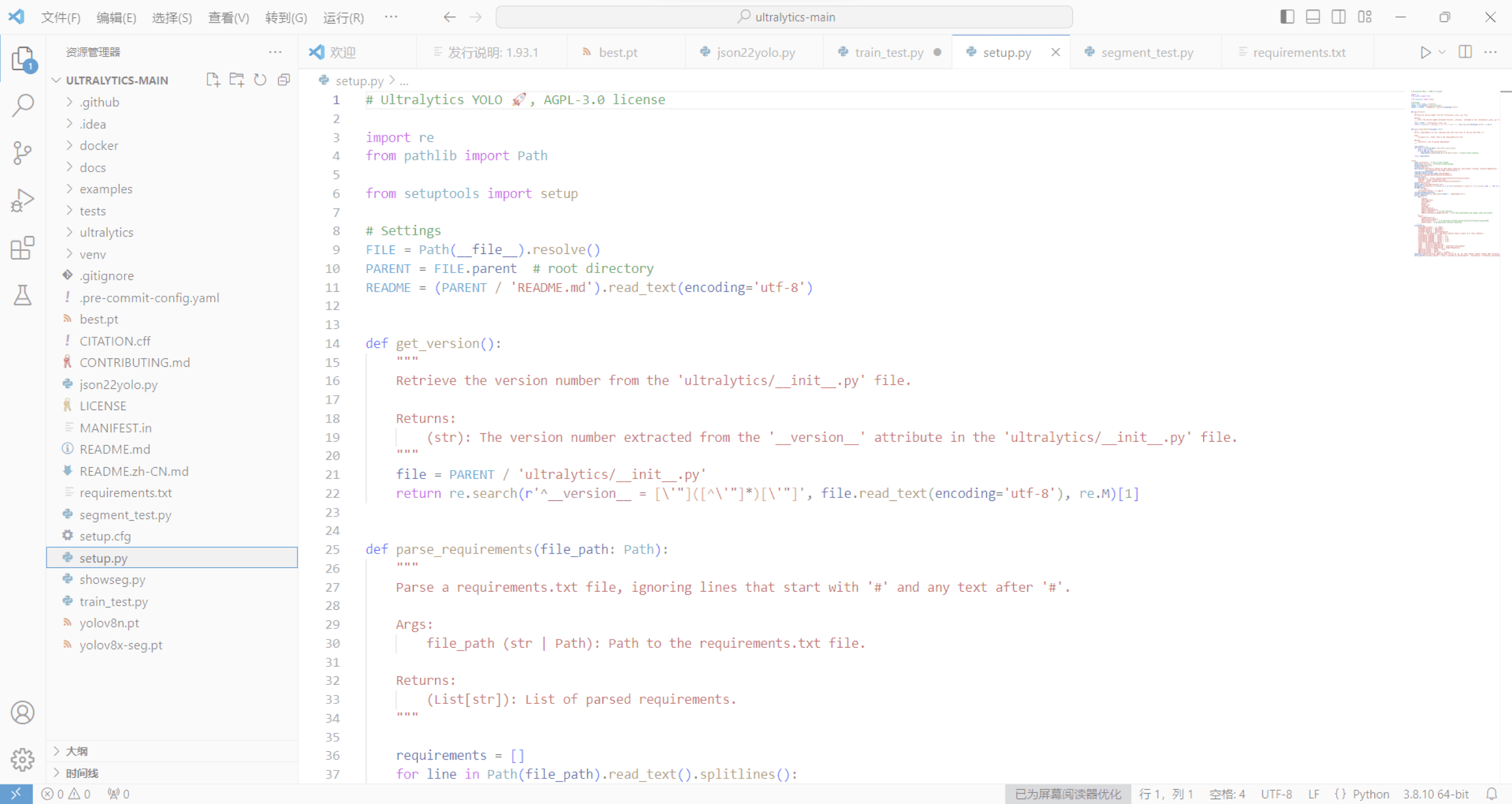
Ulva, also known as sea lettuce, is a type of green algae that frequently grows on the surface of lakes. While it is a natural part of the aquatic ecosystem, excessive growth can lead to significant problems, including water quality degradation, disruption of local ecosystems, and complications in recreational water use.
This project was developed to help the agricultural department identify areas of Ulva growth more efficiently by using drone footage and machine learning.
The program highlights the affected areas, allowing cleanup crews to target their efforts more effectively. The goal was to streamline the process of Ulva identification, improving both the speed and accuracy of detection, and ultimately making the removal efforts more focused and cost-efficient.
Initially, I encountered two significant challenges with the project.
First, the existing program's output was unsatisfactory, with a low accuracy rate in identifying Ulva. This required us to investigate the underlying causes of these inaccuracies, including potential flaws in the training data and algorithm performance.
After identifying the issues, my mentor and I conducted a series of vision workshops to address them. We established tiered scopes for the project and thoroughly explored each functional area. I revised the design team's timeline to account for scope changes and additional contributors. By forecasting future sprints, I ensured the team stayed on track while managing workloads effectively.
In the end, we gained two key insights:
The dataset was insufficient, but due to tight deadlines, we opted to use data augmentation techniques to expand it.
Additionally, the workflow was too complex, so we simplified it by merging certain steps to improve efficiency.
Through careful tuning of the YOLOv8 model, our project was able to accurately detect and highlight Ulva on lake surfaces, reducing manual labor and enabling faster, more efficient cleanup operations. This result not only optimized time and resources but also contributed to environmental conservation efforts by maintaining cleaner, healthier water ecosystems.
I realized that this role is an extension of UX design, requiring strong leadership and the ability to oversee the entire project from a higher perspective.
low accuracy and complex workflow
Planned thoroughly, held meetings, prioritized tasks
Achieved 80% accuracy, significantly improved Ulva cleanup efficiency
Learned communication, time management, and technical understanding for leadership
Challenge
Approach
Outcome
Agricultural department
5 months: 2024 Mar. - 2024 Jul.
Project manager intern
User
Duration
My Role
© Yilin Chen 2024. Proudly hand created.
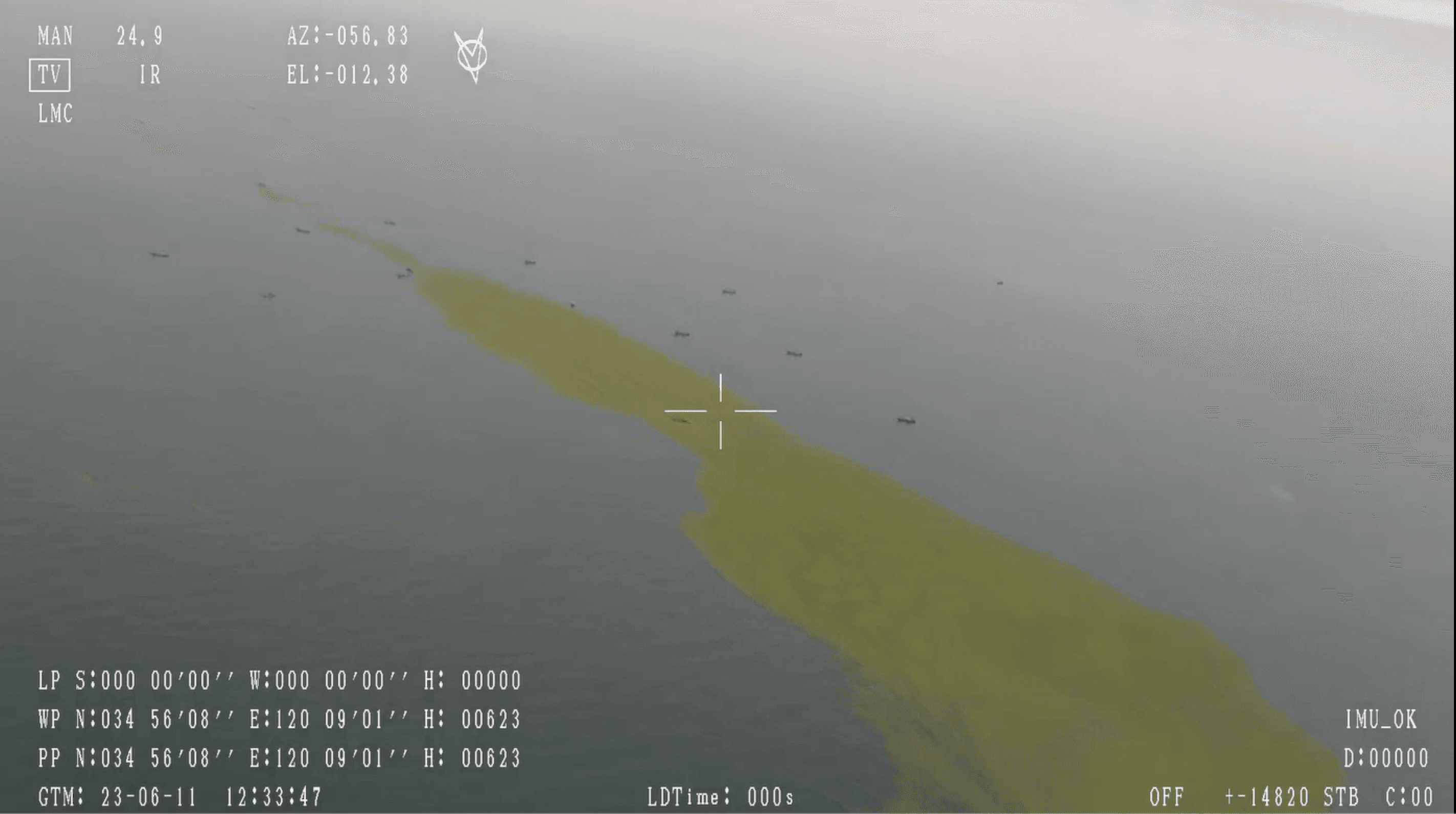
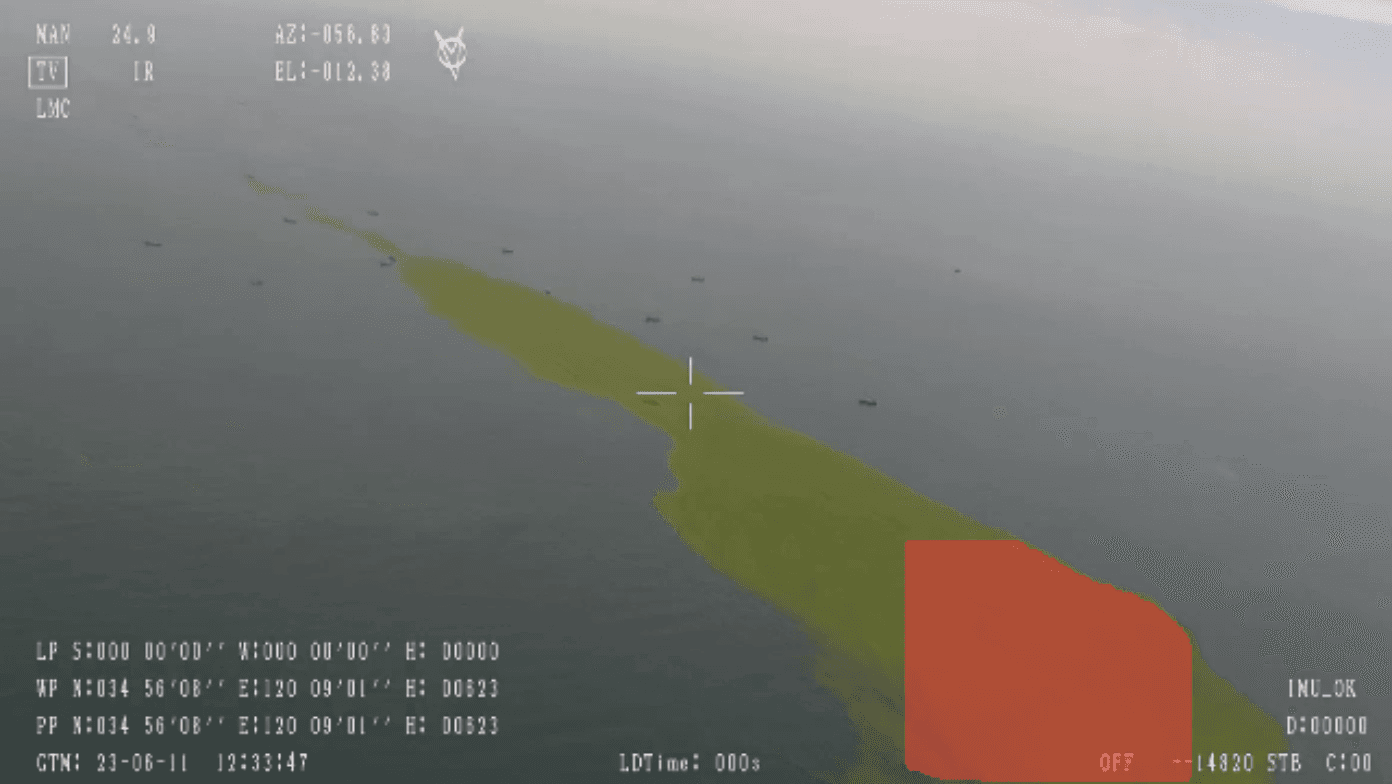
Input
Output
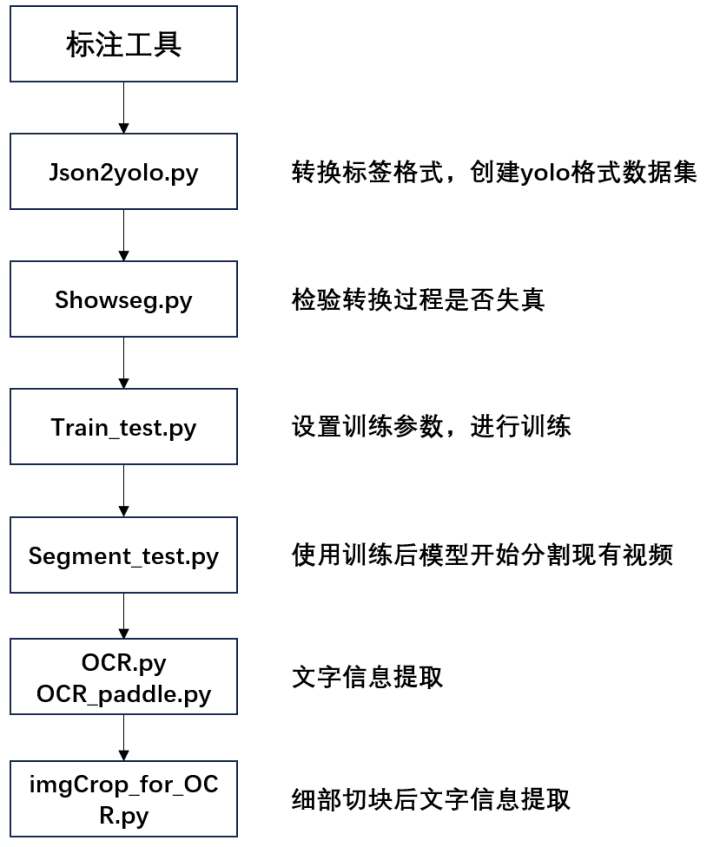
Too many files to find and excute, caused misunderstanding and mistakes among team members
Second, the overall workflow was overly complex, making it easy for team members to misunderstand their roles or make mistakes during the process. Simplifying the workflow was crucial to improving efficiency and ensuring that everyone was aligned. Addressing these issues involved close collaboration with the development team, iterating on the algorithms, and refining our processes to enhance both accuracy and usability.
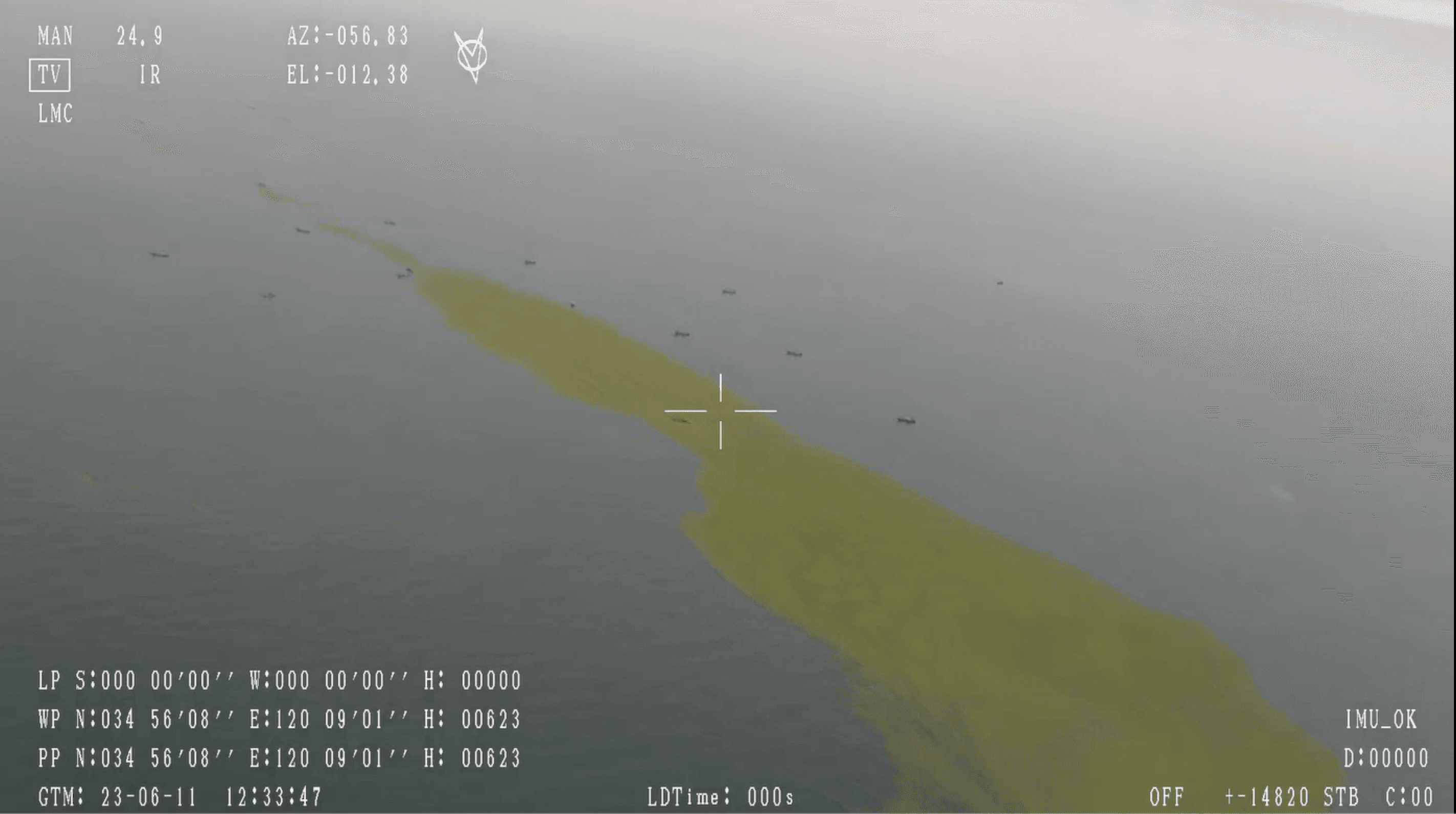
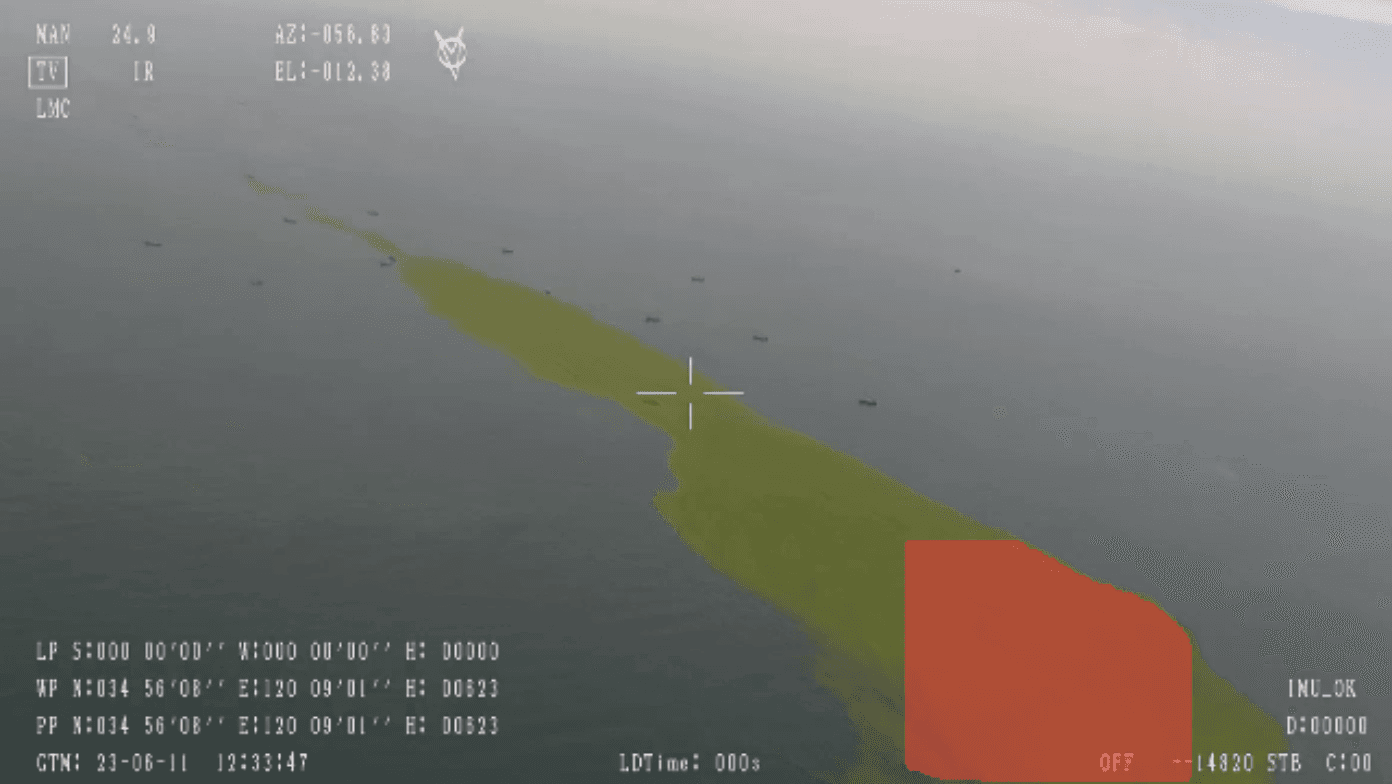
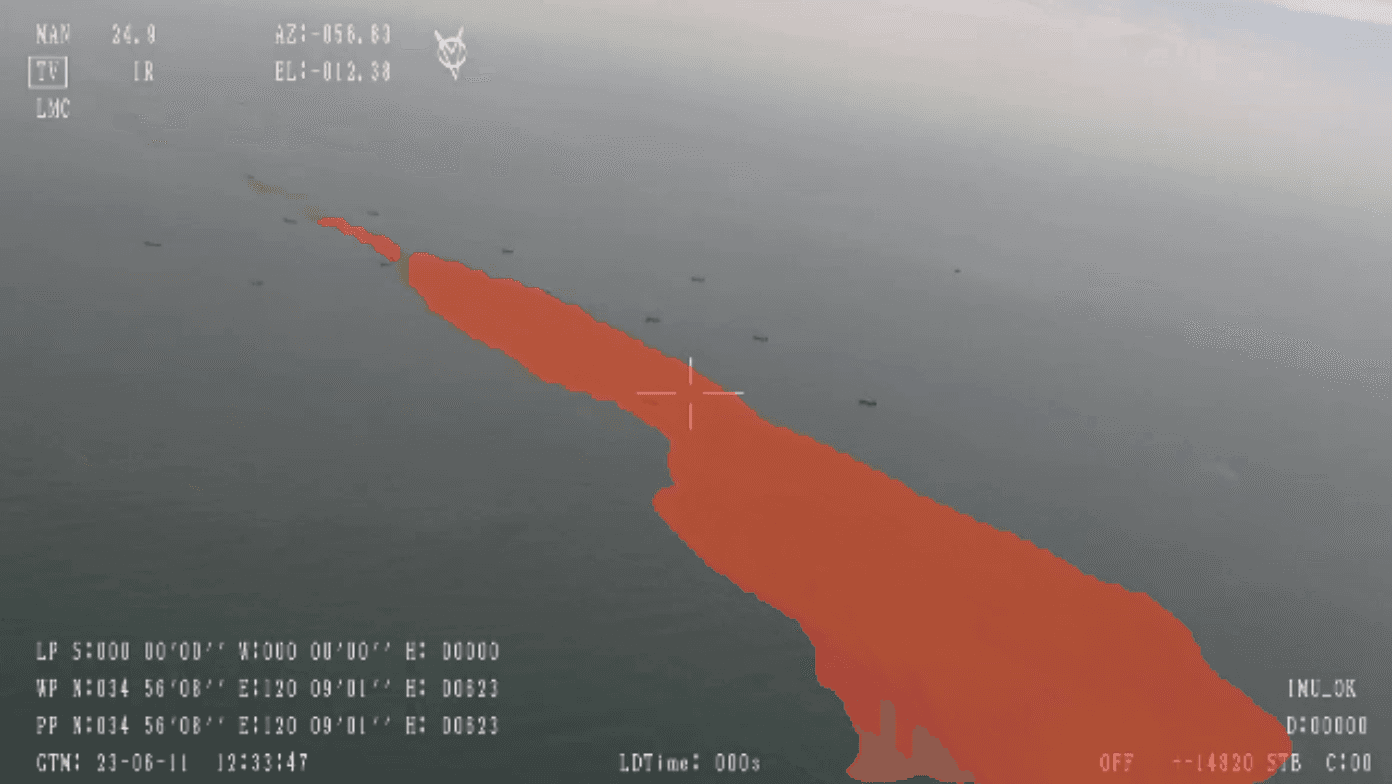
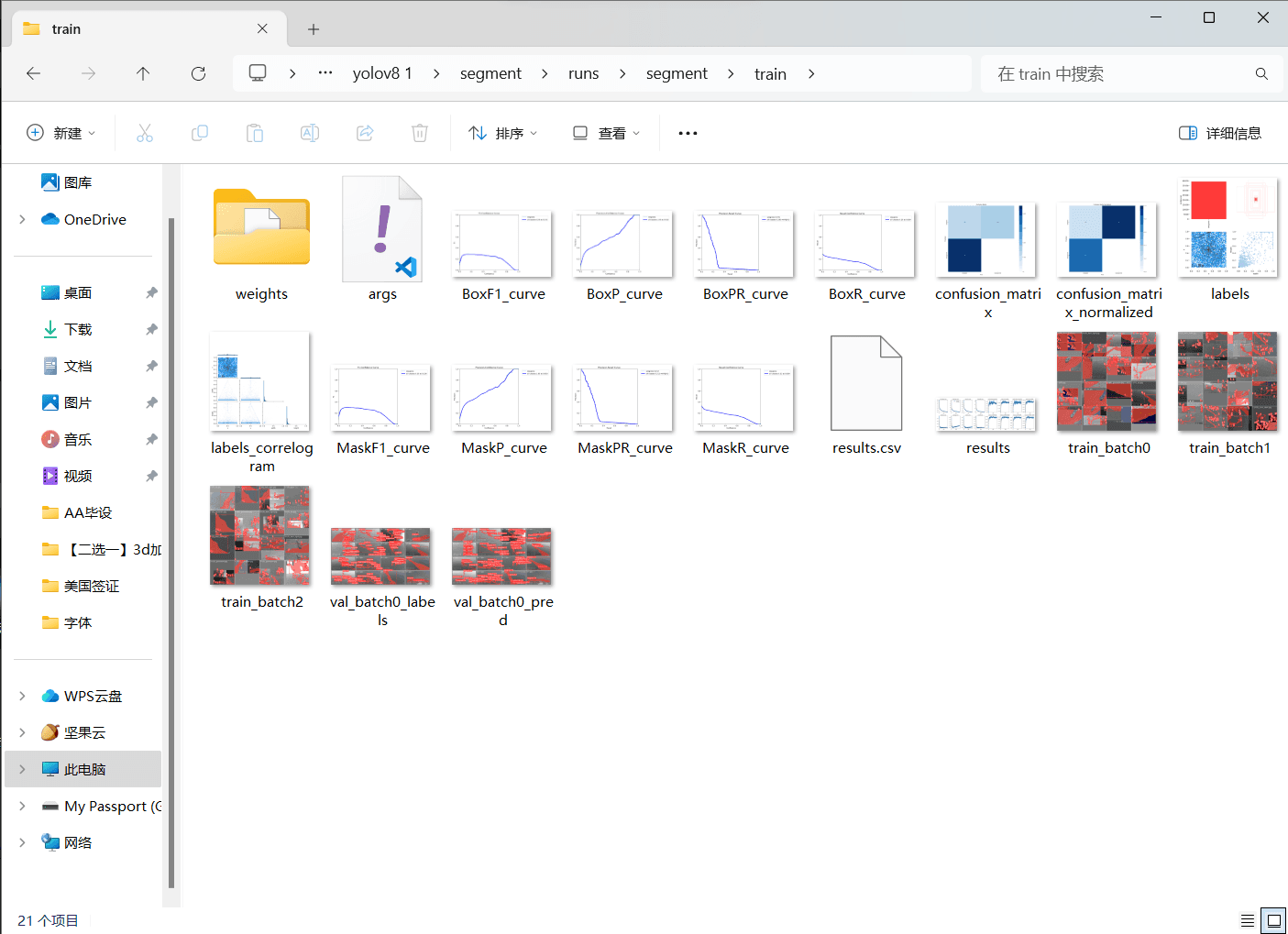
Input
Output Before
Output After
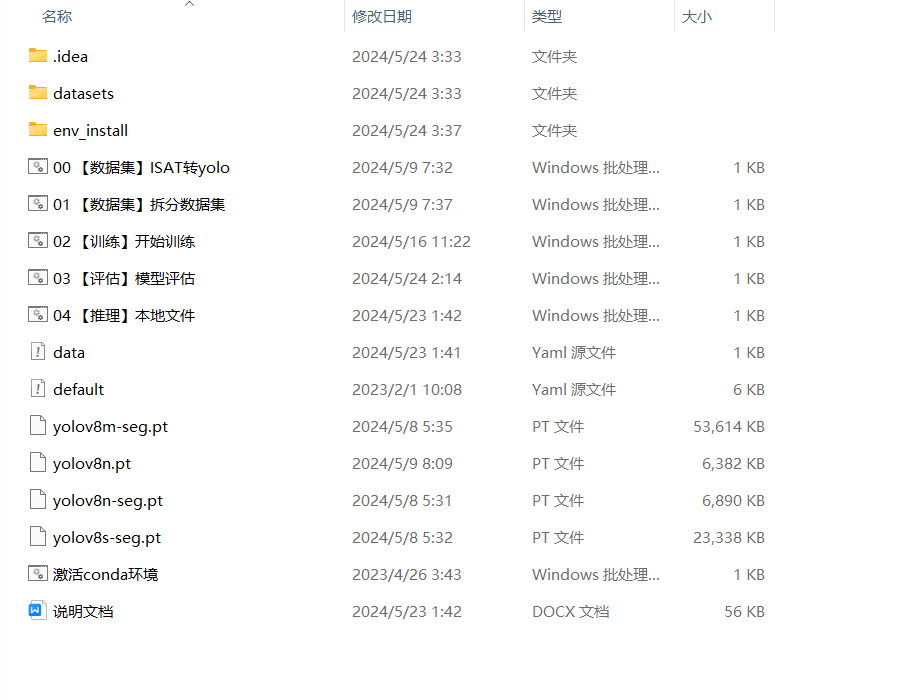
Improve the accuracy by check the evaulation and add more data by data augmentation
Simplify the process by five steps
An exiting internship with management skills to solve problems
Reflection
Project overview